Big data refers to an obtrusive and complex mass of information that is beyond the amount that one person or a small group can process.
In this article, we will look at Big Data in detail and will try to answer all of your questions with regards to Big Data.
So let’s begin!
What is Big Data?
Big Data is a term to describe the ever-increasing volume and rate of data generated by various digital devices.
This information, along with other analytics, can be used to help businesses and those in other industries make more informed decisions about customer preferences, projection for business trends, and other such topics.
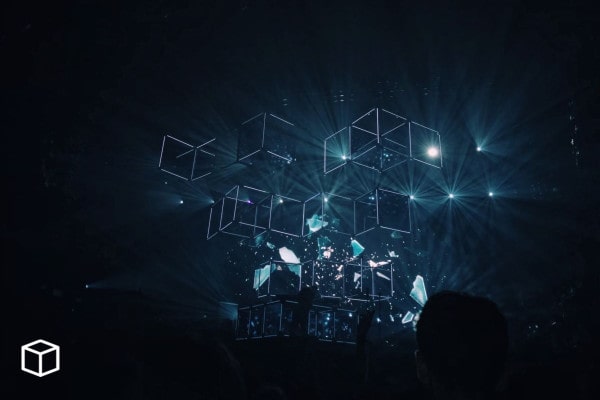
The ability of the human brain to process information from our five senses is limited. For example, just imagine if you have a comic book that is comic book in size.
If you take one page every day and read that page, it will take you nearly 30 years to complete reading that whole book. That’s how big your data generated by these digital devices is.
In today’s world, where almost every business process depends upon some sort of data analytics or the other, it is very important for professionals from various fields to know how to work with Big Data.
How is Big Data used?
Big Data is available to help us use information about customers in exciting ways.
For product development, big data could provide insights into the needs and trends in our customer base, so we can develop products in tune with their preferences.
It may also be used for competitive intelligence by providing insight into what players in the game are up to, or it might even track aggregate trends within an industry that show how health care costs are changing over time.
What are Big Data Examples?
There are many examples of big data. One example is Google’s self-driving car. Another would be Facebook’s automatic translation service, which changes the meaning of text by understanding natural languages. Such predictions make it possible to forecast how people will behave in the future.
How Big Data works?
Big data is a huge area of information that are collected by organizations around the world. Organizations collect this data for various reasons.
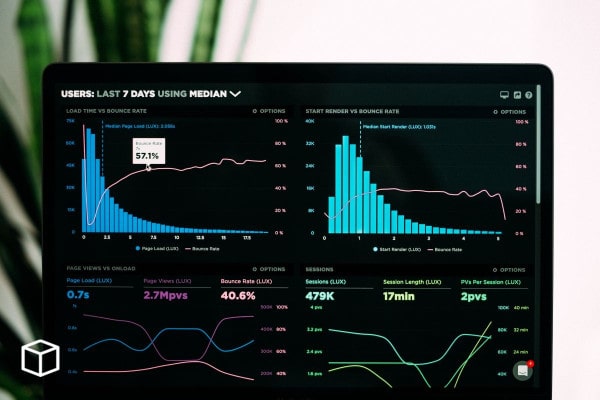
For example, if Target wants to know what clothes people are buying at their store, they gather store data and they may also look at the Facebook pages of the items that people are liking or they may search social media sites where people talk about fashion trends.
This is how they will get the big data about clothes shopping.
What is Big Data Analytics?
Big Data Analytics is the use of data analytics to extract meaning out of large datasets. It is also called “Advanced analytics” or “Extracting intelligence”.
It is an interdisciplinary subfield of computer science involving statistics, mathematics & probability theory, signal processing, pattern recognition and data management.
Big Data Analytics can be used in many different areas like marketing, fraud detection, customer analytics etc.
It provides insights that were previously impossible or prohibitively expensive to obtain using traditional techniques.
What are the Challenges of Big Data?
The challenges of Big Data are numerous. One challenge is that the data has become increasingly complex. This is due to how it is generated, its volume, and variety.
Organizations have also found that they don’t have enough storage capacity to keep this data for long periods of time. Another challenge that organizations have come across with Big Data is their inability to analyze it.
This means that there isn’t enough analysts being able to parse through the vast amounts of data available to them in order to get anything meaningful or actionable from it. This, in turn, means that there isn’t enough actionable intelligence being obtained from Big Data.
Organizations then have to wait for the data to grow bigger and wait again for analysts to be able to analyze it before they can get anything meaningful or actionable out of it. This process takes too long and slows an organization’s ability to act on data.
A possible solution to the Big Data challenges is to store it, not indefinitely but for as long as necessary. This would remove the need for analysts to constantly parse through the same data over and over again.
It is also feasible since there are now technologies that can help manage Big Data. The cost of storing this data indefinitely may be too costly but storing it for as long as necessary shouldn’t be that expensive.
This leaves us with the question of how to store Big Data. A possible solution would be to use object storage systems.
Object storage systems are designed for storing large numbers of objects not files. These objects can then be analyzed by using integrated MapReduce algorithms which can process petabytes of data with thousands of servers.
Storing Big Data in object storage systems is the perfect solution for organizations that are looking to store petabytes of data not files, whether they’re unstructured or structured.
History of Big Data?
Big data is a term used to describe the many new forms of data – both structured and unstructured. Big data itself is not a new phenomenon, but it has been driven forward by the advances in technology that have made it possible to capture more data, store huge amounts of information for extended periods, and process large datasets quickly.
The English philosopher Francis Bacon was one of the earliest people on record who described a form of “big data” when he talked about needing an automated system to collect, summarize, and correlate masses of geographical, scientific, commercial, and other textual information from libraries all over Europe. In 1946, Norbert Wiener introduced his theory of cybernetics–a study of communication and control mechanisms in organisms, machines, and organizations. A year later in 1947, Dr. Walter Shewhart at Bell Laboratories invented the Deming control chart – a statistical tool used to determine when a process required adjustment or repair.
In 1956, IBM scientist Thomas J. Watson wrote about the prospect of an automated business data processing system which provides information on all businesses listed in the US telephone book.
In 1965, Dr. Robert Fano, Massachusetts Institute of Technology (MIT), noted that information now being collected from all over the world was a new form of data. He called it “bigness.”
In 1983, Professor E.F. Codd at IBM research center gave a lecture in France on the theory of data reference where he pointed out that information could be held in databases and analyzed, something which had not been possible before.
In 1986, Dr. Clifford Lynch also at MIT wrote about the potential for finding new correlations in gigabytes (one billion bytes) of scientific data gathered by supercomputers.
What we now call big data has been around for some time. But new technologies and the so-called “three V’s” of volume, velocity, and variety – have made it more valuable than ever before.
In 2009, Doug Laney from Gartner defined three kinds of big data: 3Vs (volume, velocity, variety), 4Vs (3Vs + veracity), and 5Vs (4Vs + value).
In 2011, IBM predicted that one-to-three percent of all global data would be stored but not analyzed. That percentage has grown significantly since then.
What are the Types of Big Data?
There are two types of big data: structured and unstructured. Structured data is made up of information that’s been organized in a way that it can be easily searched, while unstructured data is made up of words or phrases that don’t have a specific order.
Unstructured data may contain a lot more valuable information than structured data, because there’s a lot more to find in it. It’s also usually easier to find deep insights from the content within the data.
Structured data may better for analyzing trends and predicting future events, but it can’t match the depth or variety of insights you’ll get from the big data you collect from unstructured sources.
What are the Three Vs of Big Data?
The 3 Vs when referring to big data are volume, velocity, and variety. The three Vs refer to three different aspects of the development of big data in an organization.
Volume is usually the easiest of the three Vs to understand. Volume refers to how much data is available for analysis.
For example, your typical Data Analyst can work with 20GB of data at once without having to worry about it slowing down the performance of their computer.
If there were 2TB (2000GB) on one hard drive, this would be the volume aspect of the three Vs. To put that into perspective, two terabytes is enough space to store about 500 Blu-ray Movies at high quality or 25,000 MP3 files.
The velocity is a term used when referring to how quickly data comes in and how fast it needs to be crunched for analysis.
Velocity in the context of big data is when you need to process large amounts of data very quickly. This usually means that your system needs to be upgraded or replaced with a larger machine, which can handle all of this crunching at once.
Finally, variety refers to how many different types of data are available for analysis. Do you have demographic, financial, and transactional data?
This could be cataloging different types of products you sell or the different customers your company interacts with. Variety in this context means having a large number of categories for an analysis to take place.
Why is Big Data important?
Big Data is important because it collects information about every person’s lifestyle, habits, actions and emotions.
Big Data is collecting raw data in various formats from diverse sources to produce actionable intelligence.
Its goal is to better predict events through analysis of patterns within the data, without relying on incomplete or low-grade information that could lead to incorrect conclusions.
What are Big Data Use Cases?
Big data use cases refer to a wide range of ways in which different organizations have been utilizing big data. Some of the ways in which organizations have been using big data include:
● Increasing the efficiency of supply chains by using customer demand prediction models
● Increasing productivity by improving machine-to-machine connectivity and real-time monitoring
● Providing cross-domain discovery of relevant insights from huge datasets to increase customer engagement and retention
● Augmenting business decision-making by building predictive models to make better business decisions
● Increasing customer satisfaction with data visualization and real-time feedback
Nowadays, big data is being extensively used for various applications. Some of the major use cases of big data include:
1. Supply Chain Management (SCM) – The SCM application of big data is one of the most common and widely used use cases. It enables organizations to gain an understanding of product demand, monitor various types of supply chains and benchmark performance metrics such as order-to-cash cycle speed, delivery time and cost optimization.
2. Retail – With advancements in technology, retailers can now gather information about customers’ shopping behavior and preferences. They can then personalize the shopping experience by offering discounts, coupons or loyalty rewards based on their spending habits.
3. Healthcare – Big data is extensively used in healthcare to manage records of patients, monitor epidemics and outbreaks, prevent hospital infections and so on. Apart from this, it can also be used to predict medical outcomes and bring about evidence-based healthcare systems. In the future, big data will play a major role in automation of tasks such as diagnostics and drug discovery/repurposing.
4. Social Media – The social media application of big data involves using it for user profiling, sentiment analysis, targeted marketing and so on. For example, Facebook once used big data to identify teenagers at their most vulnerable point and offered them help before they could even ask for it.
5. Cyber Security – Big data is playing a crucial role in cyber security by enabling the creation of systems that can detect and prevent attacks earlier than traditional security measures such as firewalls, hardware security modules or digital signatures.
6. Finance – In the finance industry as well, big data is being extensively used to gain new insights from customer behavior and improve business outcomes such as risk management and pricing. Big data is also playing a crucial role in automated trading systems where it helps provide high-speed access to large amounts of market data and execute trades.
7. Energy – Big data is also extensively used in the energy sector to improve energy efficiency, monitoring real-time demand for power, predicting outages and optimizing maintenance schedules. It can also be used for applications such as smart metering of appliances, intelligent grid management systems, demand response programs, etc.
8. Transportation – Big data is being extensively used in the transportation sector to improve traffic flow and increase road safety. It can also be used for vessel tracking, automated shipping systems, smart metering of vehicles, etc. In fact, big data has been helping national governments to reduce congestion and greenhouse gas emissions by 20 percent or more!
9. Public Safety – Big data is being extensively used in the public safety sector to improve emergency management and disaster response. It can also be used for real-time analysis of threats, early detection and prediction of epidemics/pandemics and so on.
10. Manufacturing – Big data is being increasingly used in manufacturing industries such as oil and gas, mining, aircraft manufacturing, etc. Big data can be used for predictive maintenance tasks such as detecting imminent failures in equipment by analyzing large amounts of sensor data about the current operating conditions of these equipments.
11. Education – The education industry is also increasingly using big data to monitor students’ performance and prevent drop-outs. It can also be used to develop personalized learning paths and provide better course recommendations to students.
What are some Big Data Tools?
The best Big Data tools are Hadoop, Apache Spark, Azure HDInsight, Amazon Redshift, Amazon Athena.
Hadoop is a framework that allows for whole-petabyte scale parallel data processing across clusters of computers.
Apache Spark provides a fast, in-memory cluster computing engine that can process data in real time and on disk.
Azure HDInsight makes Hadoop faster and easier to use on Windows and Linux in the cloud.
Amazon Redshift is a petabyte-scale server less data warehouse service with built in intelligence and auto-scaling that eliminates the infrastructure complexity.
At last, Amazon Athena lets interactive queries be run against datasets residing in S3 at scale when combined with Presto execution engine.
You Might Also Like
- What is Proxy server and How does it work?
- What is Hadoop and How does Hadoop work?
- What is Django and How does it work?
- What is TensorFlow and How does it work?
- What is Scala and How does it work?
- What is GitHub and how does it work?
- What is Machine Learning and how does it work?
- What is Artificial Intelligence and how does it work?
- What is Open Source Software and how does it work?
- What is a Domain Name and how does it work?